The Applications and Technical Fundamentals of OPT Deep Learning Software (SciDeepVision)
Deep Learning Software (SciDeepVision)
Automatic Feature Extraction
Deep Learning is the core technology of artificial intelligence (AI). By applying Deep Learning to image processing systems, a production machine is equipped with analysis and learning capabilities similar to humans. The machine can perform tasks such as recognizing various data information, i.e., text and images, as well as other complex pattern recognition problems.
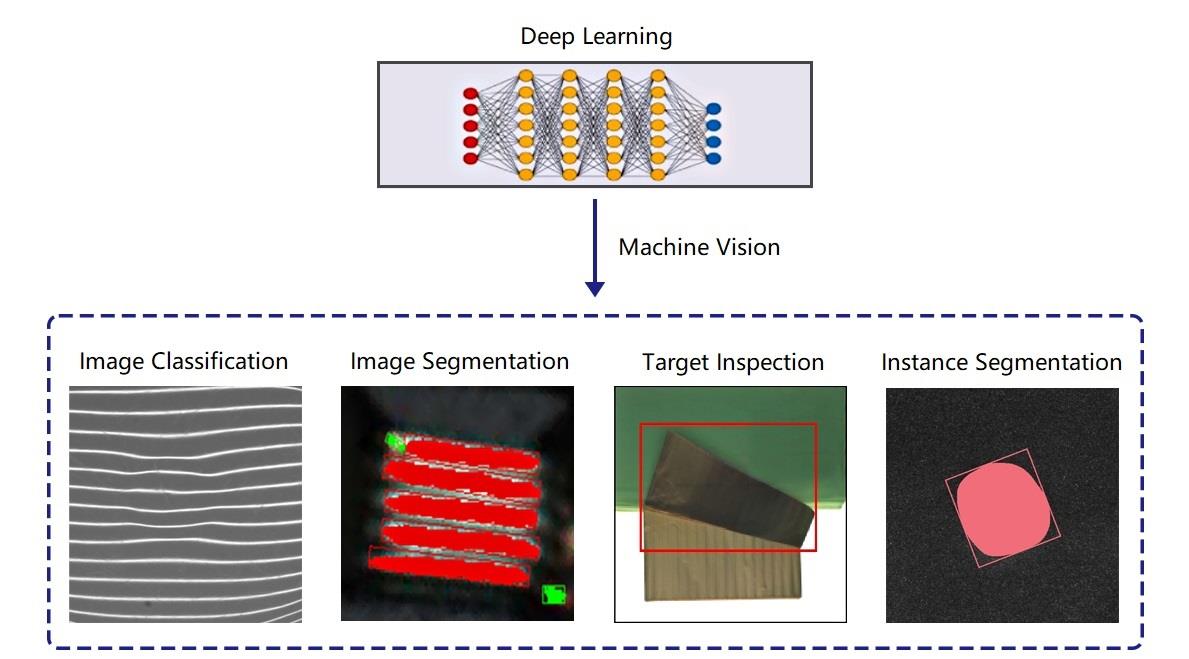
Application of Deep Learning in Machine Vision
The three most important elements of Deep Learning are algorithms, computing power and data. Machine vision applications require high computational power and large amounts of data. In this context, deep learning applications usually encounter problems such as insufficient and inadequate sample data and a large dependency on hardware performance. This affects the efficiency and accuracy of image analysis.
The SciDeepVision deep learning software, introduced by OPT, bridges the bottleneck of traditional deep learning by integrating labeling, training and evaluation. It is easy to use and requires no programming skills. It automatically extracts features and includes more than 20 key techniques and functions. It also covers labeling regularization, AI base model, model performance improvement, model training, visualization, etc.
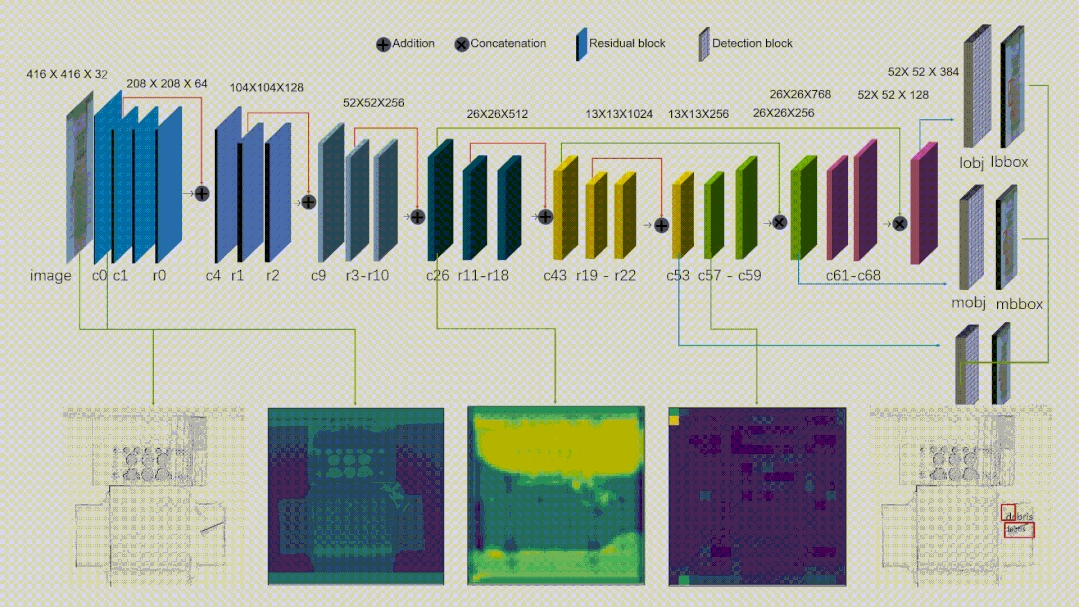
Process Visualization of the Deep Learning Algorithm
Integration of Small Sample Learning and Transfer Learning
Reduction of Dependency on Data
SciDeepVision software has achieved important technological innovations in image analysis, such as anomaly detection, text recognition, small sample learning, transfer learning, and simplified models. For example, it solves the problems of over-reliance on a large number of data from defective samples and low performance of hardware devices. The AI recognition model is more robust and saves a lot of time and manpower.
SciDeepVision software uses an abnormal defect inspection algorithm that requires only good samples, so defect position does not need to be marked. The inspection model can automatically identify the image with defects and accurately locate the defect position. At the same time, the small text sample detection algorithm can generate high-quality text images in the format of the source text from a small number of images with text information.
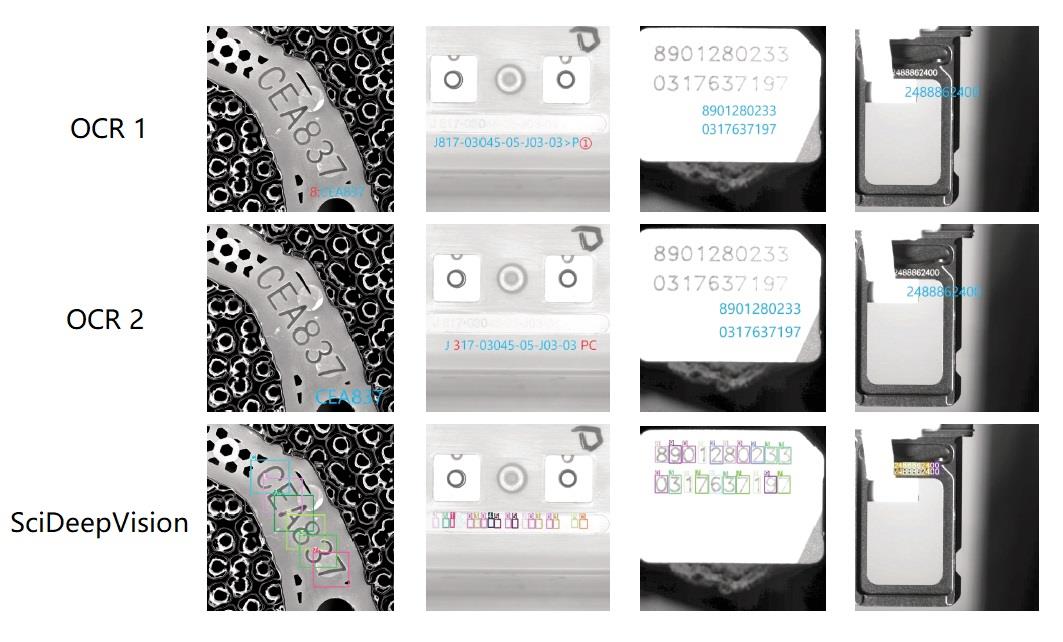
Inspection Comparison of Text Recognition Algorithms for Small Samples
When building test models, deep learning software usually has problems with robustness and poor generalizability, e.g., due to few error samples, large shape deviations, and frequent model changes. To solve such problems, OPT has developed three innovative techniques for SciDeepVision software: the small-sample learning technique, the adaptive training technique, and the transfer learning technique.
The adaptive training technique can recommend valuable data for the current inspection from previous data and manually label and optimize the model. It helps to reduce the data sorting workload by half and significantly improve the accuracy of AI model inspection at a low training cost.
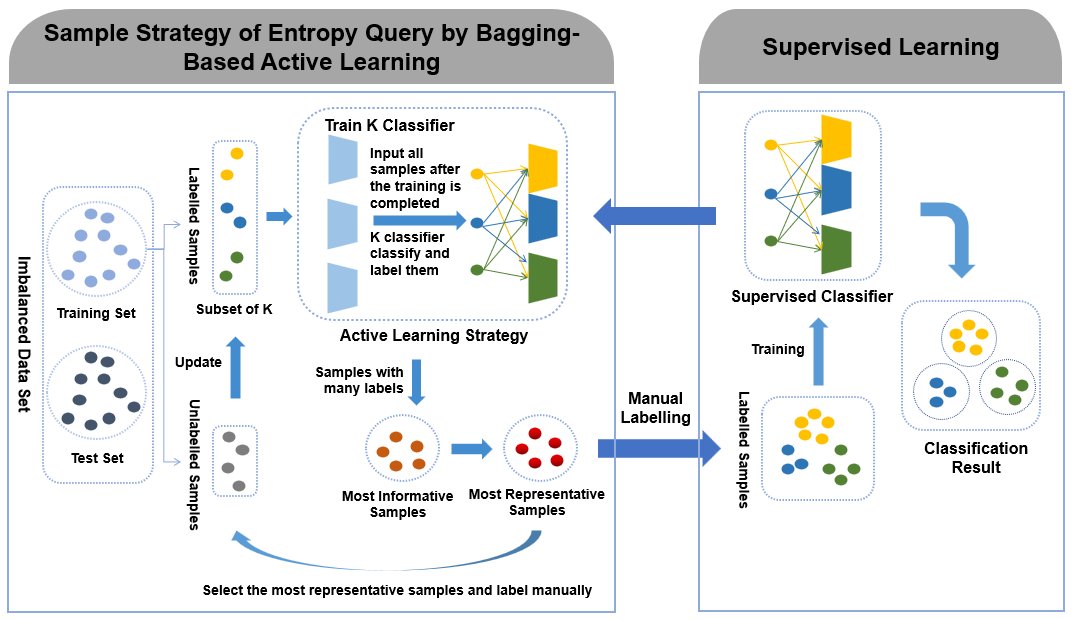
Adaptive Training Technique
The transfer learning technique of SciDeepVision software can shorten the model training cycle without compromising the robustness and generalization of the AI inspection model. The quality inspection of similar processes can even be switched with a mouse click, reducing the time for product changeover.
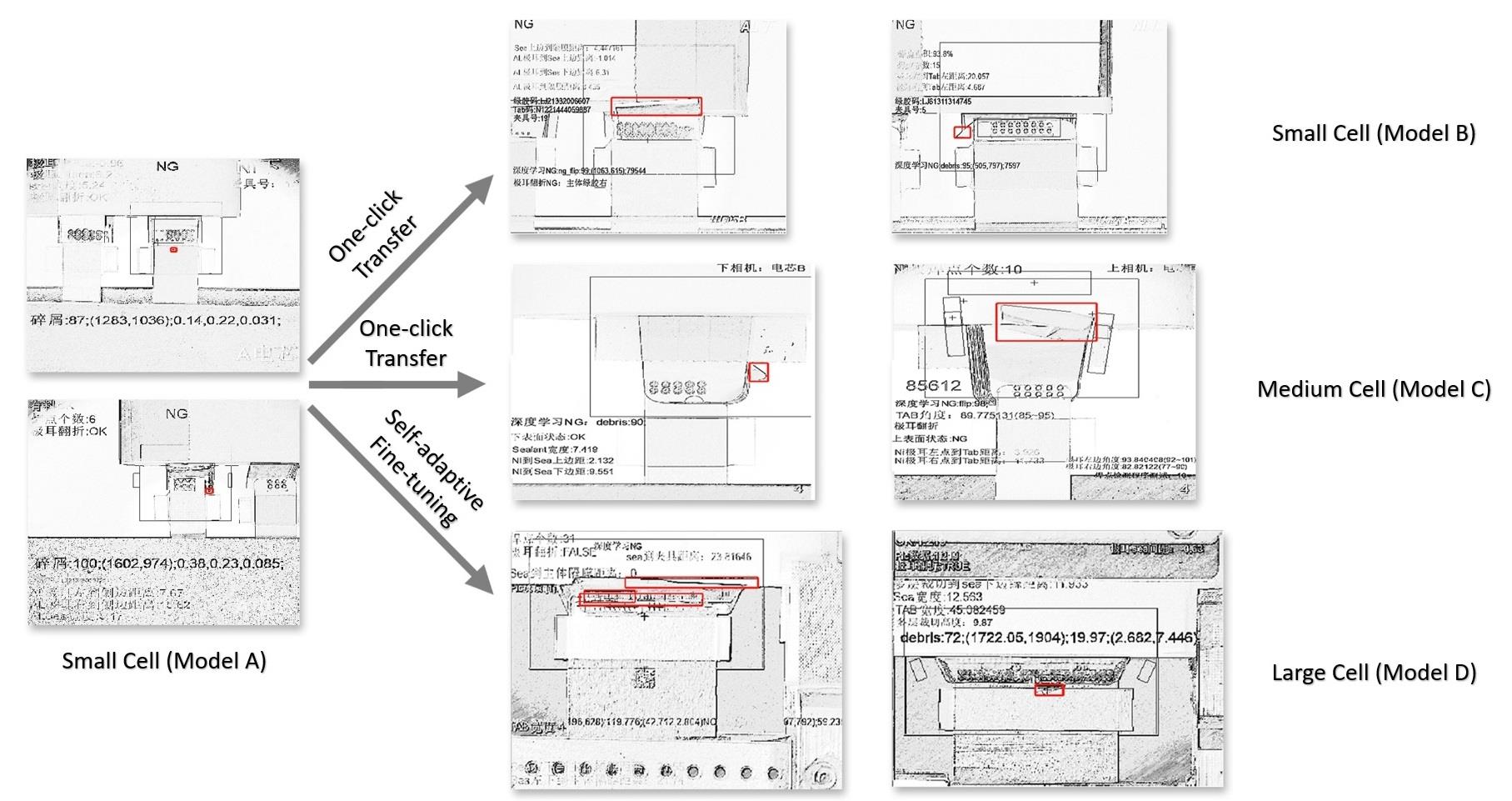
Transfer Learning Technique
Machine Vision Applications Based on Deep Learning
Covering Multiple Processes in the Production of Lithium Batteries
SciDeepVision software is widely used in industries such as lithium battery production and 3C. In lithium battery manufacturing, for example, there are all kinds and forms of external defects that cannot be detected by conventional methods. SciDeepVision software has been successfully applied to more than ten crucial processes in lithium battery manufacturing, such as coating, punching, laser cutting, stacking, winding, welding, packaging and so on.
Taking the inspection of welding defects in lithium batteries as an example, it can be seen that welding defects can have different shapes, which makes it difficult to determine their shape edges as well as random positions of defects. With the help of SciDeepVision software, convolutional neural networks can be used for feature extraction, quickly outputting information about the defect segmentation category.
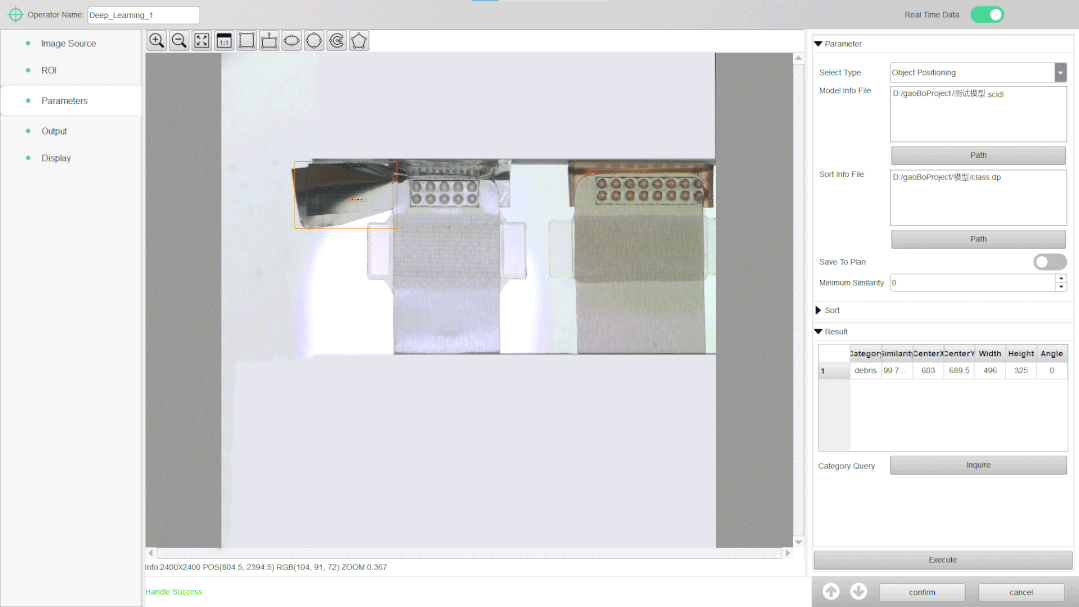
Application of the SciDeepVision Software in the Inspection of Tab Defects
Nevertheless, OPT develops a unified and user-friendly barcode positioning algorithm based on Deep Learning to enhance and correct barcode images. This enables fast and highly accurate barcode positioning with only a small amount of barcode data.